- SQL Cheat Sheet
- Background: What is SQL? Why do we need it?
- Managing Tables
- Manipulating Data
- Retrieving Attributes
- JOINS
- Subqueries
- Using Functions to Customize ResultSet
- GROUPING DATA
SQL Cheat Sheet
Background: What is SQL? Why do we need it?
This part of the Spark, Scala, and Python training includes the PySpark SQL Cheat Sheet. In this part, you will learn various aspects of PySpark SQL that are possibly asked in interviews. Also, you will have a chance to understand the most important PySpark SQL terminology.
Spark SQL uses a nested data model based on Hive It supports all major SQL data types, including boolean, integer, double, decimal, string, date, timestamp and also User Defined Data types Example of DataFrame Operations. DataFrame Operations Cont. #Access DF with DSL or SQL. Real World Problems. from pyspark.sql.types import. InferSchema sc =spark.sparkContext lines =sc.textFile('people.txt') parts = lines.map(lambda l:l.split(',')) people = parts.map(lambda p:Row(name=p0,age=int(p1))) peopledf =spark.createDataFrame(people) SpecifySchema people = parts.map(lambda p:Row(name=p0, age=int(p1.strip))). Python, or SQL (for interactive queries), and a rich set of machine learning libraries available out of the box. Compatibility with the existing Hadoop v1 (SIMR) and 2.x (YARN). Spark engine provides a way to process data in distributed memory over a cluster of.
SQL is a database language used to query and manipulate the data in the database.
Main objectives:
- To provide an efficient and convenient environment
- Manage information about users who interact with the DBMS
The SQL statements can be categorized as
Data Definition Language(DDL) Commands:
- CREATE: creates a new database object, such as a table.
- ALTER: used to modify the database object
- DROP: used to delete the objects.
Data Manipulation Language(DML) Commands:
- INSERT: used to insert a new data row record in a table.
- UPDATE: used to modify an existing record in a table.
- DELETE: used delete a record from the table.
Data Control Language(DCL) Commands:
- GRANT: used to assign permission to users to access database objects.
- REVOKE: used to deny permission to users to access database objects.
Data Query Language(DQL) Commands:
- SELECT: it is the DQL command to select data from the database.
Data Transfer Language(DTL) Commands:
- COMMIT: used to save any transaction into the database permanently.
- ROLLBACK: restores the database to the last committed state.
Identifying Data Types
Data types specify the type of data that an object can contain, such as integer data or character data. We need to specify the data type according to the data to be stored.
Following are some of the essential data types:
Data Type | Used to Store |
int | Integer data |
smallint | Integer data |
tinyint | Integer data |
bigint | Integer data |
decimal | Numeric data type with a fixed precision and scale. |
numeric | numeric data type with a fixed precision and scale. |
float | floating precision data |
money | monetary data |
datetime | data and time data |
char(n) | fixed length character data |
varchar(n) | variable length character data |
text | character string |
bit | integer data with 0 or 1 |
image | variable length binary data to store images |
real | floating precision number |
binary | fixed length binary data |
cursor | cursor reference |
sql_variant | different data types |
timestamp | unique number in the database that is updated every time in a row that contains timestamp is inserted or updated. |
table | temporary set of rows returned as a result set of a table-valued function. |
xml | store and return xml values |
Managing Tables
Create Table
Table can be created using the CREATE TABLE statement. The syntax is as follows:
Example: Create a table named EmployeeLeave in Human Resource schema with the following attributes:
Columns | Data Type | Checks |
EmployeeID | int | NOT NULL |
LeaveStartDate | date | NOT NULL |
LeaveEndDate | date | NOT NULL |
LeaveReason | varchar(100) | NOT NULL |
LeaveType | char(2) | NOT NULL |
Constraints in SQL
Constraints define rules that must be followed to maintain consistency and correctness of data. A constraint can be created by using either of the following statements:
Types of Constraints:
Constraint | Description | Syntax |
Primary key | Columns or columns that uniquely identify all rows in the table. | CREATE TABLE table_name ( col_name [CONSTRAINT constraint_name PRIMARY KEY] (col_name(s)) ) |
Unique key | Enforces uniqueness on non primary key columns. | |
Foreign key | Is used to remove the inconsistency in two tables when the data depends on other tables. | |
Check | Enforce domain integrity by restricting the values to be inserted in the column. |
3.2 Modifying Tables
Modify table using ALTER TABLE statement when:
- Adding column
- Altering data type
- Adding or removing constraints
Syntax of ALTER TABLE:
Spark Sql Examples
Renaming a Table
A table can be renamed whenever required using RENAME TABLE statement:
RENAME TABLE old_table_name TO new_table_name;
Dropping a Table versus Truncate Table
A table can be dropped or deleted when no longer required using DROP TABLE statement:
The contents of the table can be deleted when no longer required without deleting the table itself using TRUNCATE TABLE statement:
Manipulating Data
Storing Data in a Table
Syntax:
Example: Inserting data into Student table.
Example: Inserting multiple data into Student table.
Copying Data from one table to another:
Updating Data in a Table
Data can be updated in the table using UPDATE DML statement:
Example update marks of Andy to 85
Deleting Data from a Table
A row can be deleted when no longer required using DELETE DML statement.
Syntax:
Deleting all records from a table:
Retrieving Attributes
One or more column can be displayed while retrieving data from the table.
One may want to view all the details of the Employee table or might want to view few columns.
Required data can be retrieved data from the database tables by using the SELECT statement.
The syntax of SELECT statement is:
Consider the following Student table:
StudentID | FirstName | LastName | Marks |
101 | John | Ray | 78 |
102 | Steve | Jobs | 89 |
103 | Ben | Matt | 77 |
104 | Ron | Neil | 65 |
105 | Andy | Clifton | 65 |
106 | Park | Jin | 90 |
Retrieving Selected Rows
To retrieve selected rows from a table use WHERE clause in the SELECT statement.
HAVING Clause is used instead of WHERE for aggregate functions.
Comparison Operators
Comparison operators test for the similarity between two expressions.
Syntax:
Example of some comparison operators:
Logical Operators
Logical operators are used to SELECT statement to retrieve records based on one or more conditions. More than one logical operator can be combined to apply multiple search conditions.
Syntax:
Types of Logical Operators:
OR Operator
AND Operator
NOT Operator
Range Operator
Range operator retrieves data based on range.
Syntax:
Types of Range operators:
BETWEEN
NOT BETWEEN
Retrieve Records That Match a Pattern
Data from the table can be retrieved that match a specific pattern.
The LIKE keyword matches the given character string with a specific pattern.
Displaying in a Sequence
Use ORDER BY clause to display the data retrieved in a specific order.
Displaying without Duplication
The DISTINCT keyword is used to eliminate rows with duplicate values in a column.
Syntax:
JOINS
Joins are used to retrieve data from more than one table together as a part of a single result set. Two or more tables can be joined based on a common attribute.
Types of JOINS:
Consider two tables Employees and EmployeeSalary
EmployeeID (PK) | FirstName | LastName | Title |
1001 | Ron | Brent | Developer |
1002 | Alex | Matt | Manager |
1003 | Ray | Maxi | Tester |
1004 | August | Berg | Quality |
EmployeeID (FK) | Department | Salary |
1001 | Application | 65000 |
1002 | Digital Marketing | 75000 |
1003 | Web | 45000 |
1004 | Software Tools | 68000 |
INNER JOIN
An inner join retrieves records from multiple tables by using a comparison operator on a common column.

Pyspark Cheat Sheet
Syntax:
Example:
OUTER JOIN
An outer join displays the resulting set containing all the rows from one table and the matching rows from another table.
An outer join displays NULL for the column of the related table where it does not find matching records.
Syntax:
Types of Outer Join
LEFT OUTER JOIN: In left outer join all rows from the table on the left side of the LEFT OUTER JOIN keyword is returned, and the matching rows from the table specified on the right side are returned the result set.
Example:
RIGHT OUTER JOIN: In right outer join all rows from the table on the right side of the RIGHT OUTER JOIN keyword are returned, and the matching rows from the table specified on the left side are returned is the result set.
Example:
FULL OUTER JOIN: It is a combination of left outer join and right outer join. This outer join returns all the matching and non-matching rows from both tables. Whilst, the matching records are displayed only once.
Example:
CROSS JOIN
Also known as the Cartesian Product between two tables joins each row from one table with each row of another table. The rows in the result set is the count of rows in the first table times the count of rows in the second table.
Syntax:
EQUI JOIN
An Equi join is the same as inner join and joins tables with the help of foreign key except this join is used to display all columns from both tables.
SELF JOIN
In self join, a table is joined with itself. As a result, one row is in a table correlates with other rows in the same table. In this join, a table name is mentioned twice in the query. Hence, to differentiate the two instances of a single table, the table is given two aliases. Syntax:
Subqueries
An SQL statement that is used inside another SQL statement is termed as a subquery.
They are nested inside WHERE or HAVING clause of SELECT, INSERT, UPDATE and DELETE statements.
- Outer Query: Query that represents the parent query.
- Inner Query: Query that represents the subquery.
Using IN Keyword
If a subquery returns more than one value, we might execute the outer query if the values within the columns specified in the condition match any value in the result set of the subquery.
Syntax:
Using EXISTS Keyword
EXISTS clause is used with subquery to check if a set of records exists.
TRUE value is returned by the subquery in case if the subquery returns any row.
Syntax:
Using Nested Subqueries
A subquery can contain more than one subqueries. Subqueries are used when the condition of a query is dependent on the result of another query, which is, in turn, is dependent on the result of another subquery.
Syntax:
Correlated Subquery
A correlated subquery can be defined as a query that depends on the outer query for its evaluation.
Using Functions to Customize ResultSet
Various in-built functions can be used to customize the result set.
Syntax:
Using String Functions
String values in the result set can be manipulated by using string functions.
They are used with char and varchar data types.
Following are the commonly used string functions are:
Function Name | Example |
left | |
len | |
lower | |
reverse | |
right | |
space | |
str | |
substring | |
upper |
Using Date Functions
Date functions are used to manipulate date time values or to parse the date values.
Date parsing includes extracting components, such as day, month, and year from a date value.
Some of the commonly used date functions are:
Function Name | Parameters | Description |
dateadd | (date part, number, date) | Adds the number of date parts to the date. |
datediff | (date part, date1, date2) | Calculates the number of date parts between two dates. |
Datename | (date part, date) | Returns date part from the listed as a character value. |
datepart | (date part, date) | Returns date part from the listed as an integer. |
getdate | 0 | Returns current date and time |
day | (date) | Returns an integer, which represents the day. |
month | (date) | Returns an integer, which represents the month. |
year | (date) | Returns an integer, which represents the year. |
Using Mathematical Functions
Numeric values in a result set can be manipulated in using mathematical functions.
The following table lists the mathematical functions:
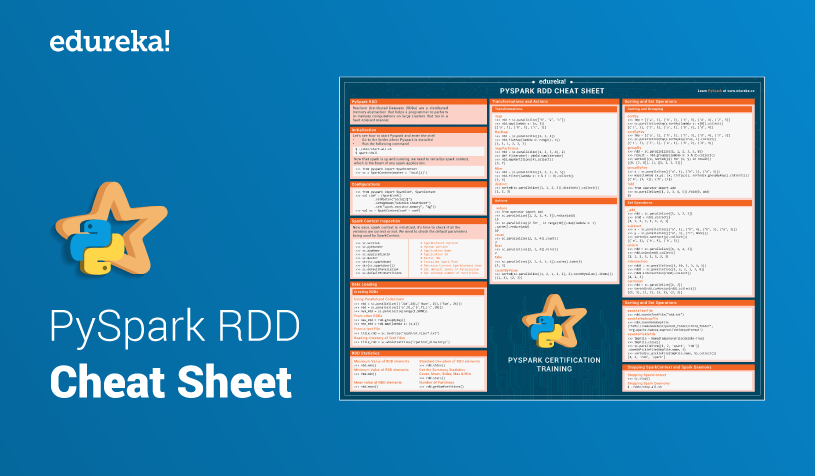
Function Name | Parameters | Description |
abs | (numeric_expression) | Returns an absolute value |
acts,asin,atan | (float_expression) | Returns an angle in radians |
cos, sin, cot,tan | (float_expression) | Returns the cosine, sine, cotangent, or tangent of the angle in radians. |
degrees | (numeric_expression) | Returns the smallest integer greater than or equal to specifies value. |
exp | (float_expression) | Returns the exponential value of the specified value. |
floor | (numeric_expression) | Returns the largest integer less than or equal to the specified value. |
log | (float_expression) | Returns the natural logarithm of the specified value. |
pi | 0 | Returns the constant value of 3.141592653589793 |
power | (numeric_expression,y) | Returns the value of numeric expression to the value of y |
radians | (numeric_expression) | Converts from degrees to radians. |
rand | ([seed]) | Returns a random float number between 0 and 1. |
round | (numeric_expression,length) | Returns a numeric expression rounded off to the length specified as an integer expression. |
sign | (numeric_expression) | Returns positive, negative or zero. |
sqrt | (float_expression) | Returns the square root of the specified value. |
Using Ranking Functions
Ranking functions are used to generate sequential numbers for each row to give a rank based on specific criteria.
Ranking functions return a ranking value for each row. Following functions are used to rank the records:
- row_number Function: This function returns the sequential numbers, starting at 1, for the rows in a result set based on a column.
- rank Function: This function returns the rank of each row in a result set based on specified criteria.
- dense_rank Function: The dense_rank() function is used where consecutive ranking values need to be given based on specified criteria.
These functions use the OVER clause that determines the ascending or descending sequence in which rows are assigned a rank.
Using Aggregate Functions
The aggregate functions, on execution, summarize the values for a column or group of columns and produce a single value.
Syntax:
Following are the aggregate functions:
Function Name | Description |
avg | returns the average of values in a numeric expression, either all or distinct. |
count | returns the number of values in an expression, either all or distinct. |
min | returns the lowest value in an expression. |
max | returns the highest value in an expression. |
sum | returns the total of values in an expression, either all or distinct. |
GROUPING DATA
Grouping data means to view data that match a specific criteria to be displayed together in the result set.
Data can be grouped by using GROUP BY, COMPUTE,COMPUTE BY and PIVOT clause in the SELECT statement.
GROUP BY Clause
Summarizes the result set into groups as defined in the query by using aggregate functions.
Syntax:
COMPUTE and COMPUTE BY Clause
This COMPUTE clause, with the SELECT statement, is used to generate summary rows by using aggregate functions in the query result.
The COMPUTE BY clause can be used to calculate summary values of the result set on a group of data.
Syntax:
PIVOT Clause
The PIVOT operator is used to transform a set of columns into values, PIVOT rotates a table-valued expression by turning the unique values from one column in the expression into multiple columns in the output.

Syntax:
People are also reading:
This page contains a bunch of spark pipeline transformation methods, whichwe can use for different problems. Use this as a quick cheat on how we cando particular operation on spark dataframe or pyspark.
This code snippets are tested on spark-2.4.x version, mostly work onspark-2.3.x also, but not sure about older versions. |
Read the partitioned json files from disk
applicable to all types of files supported
Save partitioned files into a single file.
Here we are merging all the partitions into one file and dumping it intothe disk, this happens at the driver node, so be careful with sie ofdata set that you are dealing with. Otherwise, the driver node may go out of memory.
Use coalesce
method to adjust the partition size of RDD based on our needs.
Filter rows which meet particular criteria
Map with case class
Use case class if you want to map on multiple columns with a complexdata structure.
OR using Row
class.
Use selectExpr to access inner attributes
Provide easily access the nested data structures like json
and filter themusing any existing udfs, or use your udf to get more flexibility here.
How to access RDD methods from pyspark side
Using standard RDD
operation via pyspark API isn’t straight forward, to get thatwe need to invoke the .rdd
to convert the DataFrame to support these features.
For example, here we are converting a sparse vector to dense and summing it in column-wise.
Pyspark Map on multiple columns
Filtering a DataFrame column of type Seq[String]
Filter a column with custom regex and udf
Sum a column elements
Remove Unicode characters from tokens
Sometimes we only need to work with the ascii text, so it’s better to clean outother chars.
Connecting to jdbc with partition by integer column
When using the spark to read data from the SQL database and then do theother pipeline processing on it, it’s recommended to partition the dataaccording to the natural segments in the data, or at least on an integercolumn, so that spark can fire multiple sql queries to read data from SQLserver and operate on it separately, the results are going to the sparkpartition.
Bellow commands are in pyspark, but the APIs are the same for the scala version also.
Parse nested json data
This will be very helpful when working with pyspark
and want to pass verynested json data between JVM and Python processes. Lately spark community relay onapache arrow project to avoid multiple serialization/deserialization costs whensending data from java memory to python memory or vice versa.
So to process the inner objects you can make use of this getItem
methodto filter out required parts of the object and pass it over to python memory viaarrow. In the future arrow might support arbitrarily nested data, but right now it won’tsupport complex nested formats. The general recommended option is to go without nesting.
'string ⇒ array<string>' conversion
Type annotation .as[String]
avoid implicit conversion assumed.
Spark Sql Cheat Sheet
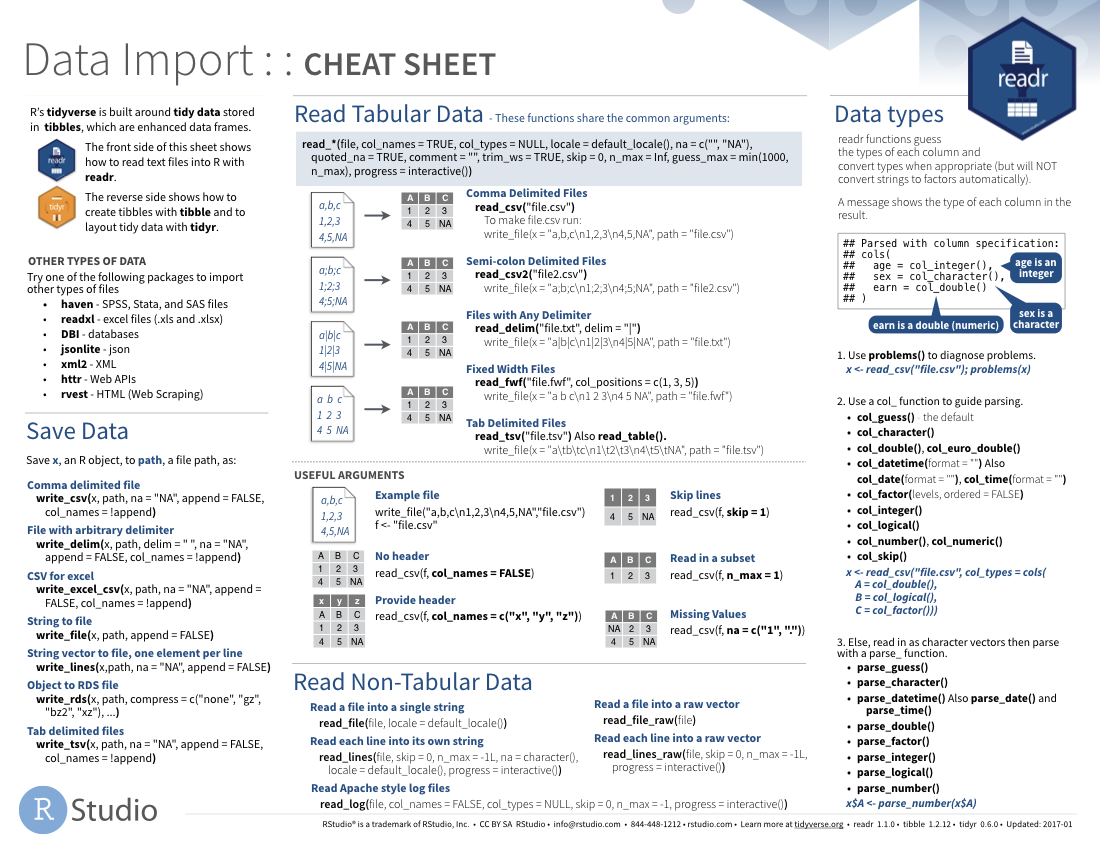
A crazy string collection and groupby
This is a stream of operation on a column of type Array[String]
and collectthe tokens and count the n-gram distribution over all the tokens.
How to access AWS s3 on spark-shell or pyspark
Most of the time we might require a cloud storage provider like s3 / gs etc, toread and write the data for processing, very few keeps in-house hdfs to handle the datathemself, but for majority, I think cloud storage easy to start with and don’t needto bother about the size limitations.
Supply the aws credentials via environment variable
Supply the credentials via default aws ~/.aws/config file
Recent versions of awscli
expect its configurations are kept under ~/.aws/credentials
file,but old versions looks at ~/.aws/config
path, spark 2.4.x version now looks at the ~/.aws/config
locationsince spark 2.4.x comes with default hadoop jars of version 2.7.x.
Set spark scratch space or tmp directory correctly
This might require when working with a huge dataset and your machine can’t hold themall in memory for given pipeline steps, those cases the data will be spilled overto disk, and saved in tmp directory.
Set bellow properties to ensure, you have enough space in tmp location.
Pyspark doesn’t support all the data types.
When using the arrow
to transport data between jvm to python memory, the arrow may throwbellow error if the types aren’t compatible to existing converters. The fixes may becomein the future on the arrow’s project. I’m keeping this here to know that how the pyspark getsdata from jvm and what are those things can go wrong in that process.
Work with spark standalone cluster manager
Start the spark clustering in standalone mode
Once you have downloaded the same version of the spark binary across the machinesyou can start the spark master and slave processes to form the standalone sparkcluster. Or you could run both these services on the same machine also.
Standalone mode,
Worker can have multiple executors.
Worker is like a node manager in yarn.
We can set worker max core and memory usage settings.
When defining the spark application via spark-shell or so, define the executor memory and cores.
Pyspark Tutorial For Beginners Pdf
When submitting the job to get 10 executor with 1 cpu and 2gb ram each,
This page will be updated as and when I see some reusable snippet of code for spark operations |
Changelog
References
Go Top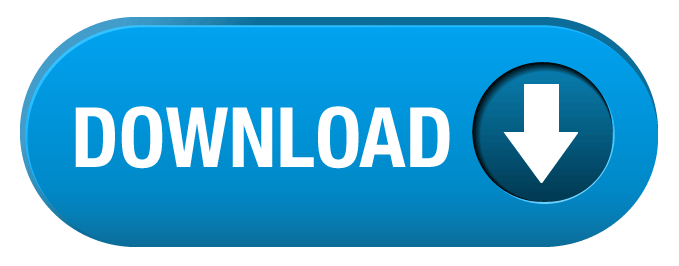